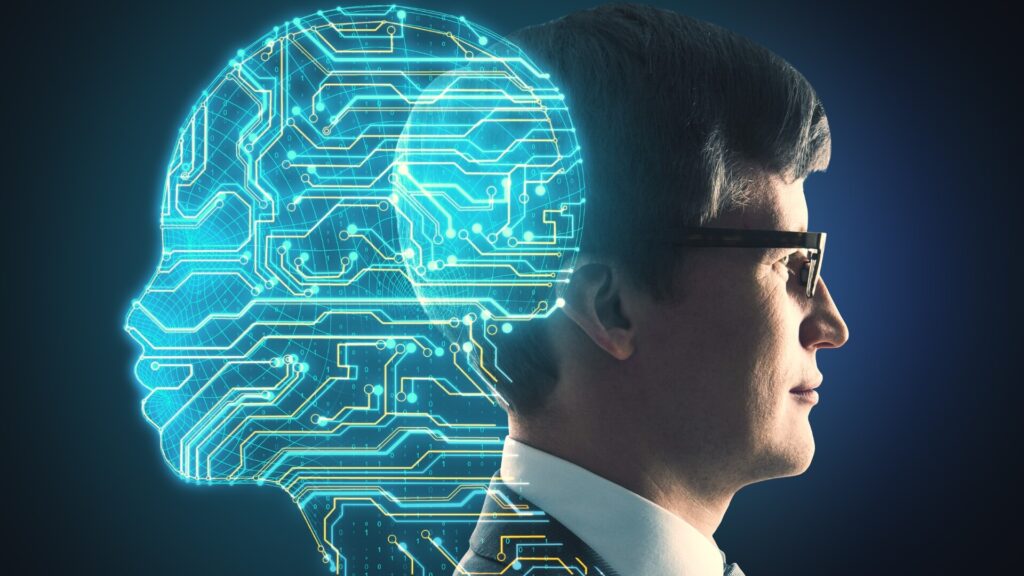
The conventional method of Credentialing and Enrollment is knowledge and effort-intensive that is not just time-consuming but prone to errors.
The Enrollment and certification process can have better outcomes by using Artificial Intelligence and Machine Learning.
AI and ML can eliminate the need for constant manual involvement using algorithms to analyze data and make decisions, and if deployed easily can save time and lower the possibility of mistakes. The following advantages are evident of using AI and ML:
- Enhanced Efficiency: AI and ML can drastically cut the time and resources needed for Enrollment and Credentialing, freeing up valuable time.
- Increased Accuracy: ML and AI can help to ensure data accuracy and completeness, as well as to help decrease errors.
- Improved Compliance: AI and ML can assist in making sure Healthcare Providers adhere to legal and regulatory obligations.
So, what are AI and ML:
- AI is the ability of computers to perform tasks that require human intelligence.
- ML is a subset of AI that allows computers to learn from data and make predictions.
- AI and ML can transform your business by automating processes, enhancing customer experience, and generating insights
AI and ML in Healthcare
- Streamlining the Credentialing and Enrollment process.
- Improving patient care
- Predicting re-admissions and complications.
- Streamlining administrative tasks
- Analyze claims data to identify instances of fraud and waste, helping to reduce Healthcare costs and improve the overall efficiency of the system.
Benefits of using AI and ML in Credentialing:
The use of artificial intelligence (AI) and machine learning (ML) in the Healthcare Industry has revolutionized the process. Here are some of the benefits:
- Precision: AI and ML systems can analyze documents and make onboarding a breeze. Can assist in minimizing errors and ensuring that primary source verification is faster.
- Efficiency gain: By using AI and ML, many of the data entry and verification procedures associated with Enrollment and Credentialing can be automated. This can free up time and lessen the workload for Healthcare Professionals, allowing them to concentrate on other crucial responsibilities.
- Improved security: By detecting potential threats and weaknesses in real time, AI and ML can be utilized to enhance the security of healthcare data.
- Enhanced compliance: Healthcare professionals are required to follow a number of rules and guidelines, and noncompliance has major repercussions. Healthcare providers can continue to be compliant with pertinent legislation and all standards can be satisfied and marked compliant 0r not instantly.
Challenges and considerations of AI and ML in Credentialing and Enrollment for Healthcare Providers
While there are many advantages to adopting AI and ML in the Credentialing process and Enrollment of Healthcare Practitioners, there are certain difficulties and factors that need to be taken into account as well. Some of the most significant are listed below:
- Integration with current systems: For AI and ML systems to be effective, they must be able to integrate components of current Healthcare Provider systems. This can be difficult because it might not be possible to do this in many legacy systems. The costs and difficulties of integrating these technologies will need consideration
- Accuracy and bias: The data that ML systems are trained on determines how good they are. In order to assure accuracy and reduce bias, it is crucial to make sure that the data utilized to train these algorithms are representative of the populations they will be applied to. Not only that manual checks need to be considered at various output points to ensure the ML training of the system is accurate and moving in the right direction.
- Legacy Data Considerations & Data Standardization The data may not be in a format that can be easily transferrable
- Before the information can be successfully incorporated into the new system, it might need to be digitalized or transformed into a format that is compatible. Examples of AI and ML in healthcare provider enrolment and credentialing
To simplify and streamline the Credentialing and Enrolling process, the Healthcare Sector has recently begun to utilize artificial intelligence (AI) and machine learning (ML) technology. Here are some instances of how AI and ML are being applied to the Enrolment and Credentialing of Medical Professionals:
Analysis of provider data and documentation using natural language processing (NLP)
NLP is a subset of AI that can examine unstructured material, including provider credentials and resumes. This technology can automatically retrieve pertinent data and analyze it for precision, thoroughness, and consistency. NLP enables Healthcare Companies to process massive volumes of provider data quickly and guarantee the accuracy and timeliness of all documentation.
Automated verification and validation of Provider Credentials
AI and ML can automate the verification and validation of provider credentials, which is a crucial part of the Credentialing process. This technology can verify education, certification, and licensing information in real-time, reducing the time and cost associated with manual verification. Automated Credentialing can also reduce errors and increase accuracy, ensuring that only qualified providers are enrolled.
Predictive analytics for fraud detection
AI and ML can be used to analyze provider data and identify patterns that may indicate fraud. Predictive analytics can detect anomalies in billing patterns, referral patterns, and prescribing practices, helping to prevent fraudulent behavior before it occurs. By detecting fraud early, healthcare organizations can save money and protect their reputation.
Conclusion
These technologies hold significant promise for enhancing precision, and effectiveness, and minimizing errors in the Credentialing and Enrollment processes. Healthcare Providers can get advantages including quicker processing times, higher accuracy, and eventually Lower costs by choosing a partner that is implementing AI and ML in their Credentialing and Enrollment processes.
Healthcare Professionals must adopt new technologies and stay on the cutting edge as Healthcare continues to change.
As a leading provider of Healthcare Services, Apaana Healthcare provides innovative solutions to address the challenges of Credentialing and Enrollment.
Our team of experts is equipped with the latest tools and technologies to streamline the Credentialing process and ensure compliance with regulations.
Don’t let the challenges of Credentialing and Enrollment hold you back. Take the first step towards a more efficient and effective Credentialing process by contacting Apaana Healthcare today.
References
- How Machine Learning in Healthcare Works and Why it Matters (2022). How Machine Learning in Healthcare Works and Why it Matters (emeritus.org)
- Suresh, H., Hunt, N., & Johnson, A. (2019). Artificial Intelligence in Healthcare Provider Credentialing and Enrollment: A Review. Healthcare Quarterly (Toronto, Ont.), 22(2), 39–44. https://doi.org/10.12927/hcq.2019.25912
- De Moor, G. (2020). Artificial Intelligence in Healthcare: Past, Present and Future. Biochemia Medica, 30(3), 030201. https://doi.org/10.11613/bm.2020.030201
- American Medical Association. (2020). Augmented Intelligence. Augmented intelligence in medicine | American Medical Association (ama-assn.org)
- National Institutes of Health. (2021). Artificial Intelligence (AI). Artificial Intelligence at NIH | Data Science at NIH
- Miller, D. D., & Brown, E. W. (2018). Artificial Intelligence in Medical Practice: The Question to the Answer? The American Journal of Medicine, 131(2), 129–133. https://doi.org/10.1016/j.amjmed.2017.09.041